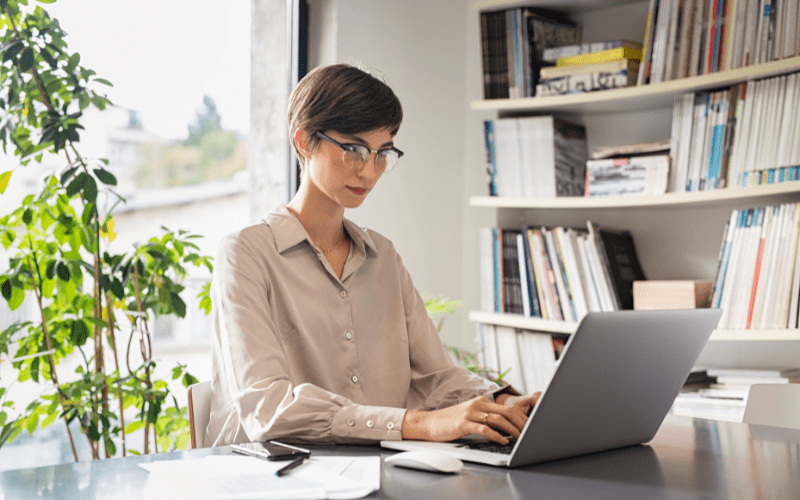
Have you ever wondered how Amazon knows exactly what you need before you even know it? Or how Google suggests such precise searches based on just a few typed letters? The answer is simple: Machine Learning.
Machine Learning, a subset of artificial intelligence (AI) is already present in many aspects of our lives, from product suggestions to weather forecasts.
Companies of all sizes are adopting this technology, recognizing its potential to boost business growth.
At Pareto, we use Machine Learning to optimize the performance of digital marketers. To find out more, read on to find out how this technology has evolved, what its benefits are and, most importantly, how it can be incorporated into your marketing strategy.
What is Machine Learning?
Machine Learning is a field of AI that focuses on developing algorithms and models that allow computer systems to learn and make decisions based on data, without being explicitly programmed for specific tasks.
The central aim of Machine Learning is to enable machines to learn from past examples or training data in order to make predictions or decisions on new, unseen data.
In this context, Machine Learning algorithms are the "brains" behind the whole process, which enables AI writing, image creation and all the new applications that are being developed. They are responsible for learning from training data and creating mathematical or statistical models that can make predictions or decisions based on new data.
The algorithm is fed the training data to learn the relationship between the inputs (data) and the desired outputs (labels or target values). After training, the model is evaluated using test data to check its accuracy and performance. This algorithm can also be run in Supervised Learning.
History and Evolution of Machine Learning
The history and evolution of Machine Learning is marked by decades of development, technological advances and innovations that have transformed the way machines can learn and make decisions based on data. Here is an overview of the history and evolution of this field:
- 1940 - 1950: The concept of Machine Learning began to be explored in the late 1940s and early 1950s. Alan Turing's pioneering work on the "Universal Machine" and the "Learning Machine" laid the theoretical foundations for the field.
- 1950 - 1960: During this time, the focus was on developing algorithms capable of performing simple machine learning tasks, such as playing chess. Arthur Samuel's chess program is a notable example of a machine learning system of the time.
- 1960 - 1970: Machine Learning was mainly associated with research into artificial intelligence. The systems were based on hand-coded rules and were unable to learn directly from the data.
- 1980 - 1990: This period saw a renewed interest in Machine Learning, especially in the areas of neural networks and automatic learning algorithms. Systems began to become more sophisticated and capable of learning from larger data sets.
- 1990 - 2000: The field witnessed significant advances in Machine Learning theory and techniques. Algorithms such as Support Vector Machines (SVMs) and Decision Trees gained popularity. The emergence of the Internet also made it more accessible to share data for training models.
- 2000 - 2010: The Big Data revolution boosted Machine Learning, as organizations began to collect and store large volumes of data. Deep learning algorithms, such as deep neural networks, began to excel at tasks such as voice recognition and computer vision.
- 2010 - 2020: Machine Learning has become a fundamental part of many products and services, including virtual assistants, recommendation systems and autonomous vehicles. Convolutional neural networks (CNNs) and recurrent neural networks (RNNs) have become popular in image processing and natural language tasks.
- 2020 - Ongoing: Machine Learning continues to evolve rapidly. Language models, such as GPT-3, and transfer learning techniques have gained prominence, allowing pre-trained models to be adjusted for specific tasks with limited data.
What Are the Most Popular Types of Machine Learning?
There are three main types of Machine Learning: Supervised Learning, Unsupervised Learning and Reinforcement Learning.
Supervised Learning
Supervised learning is a Machine Learning paradigm in which the algorithm is trained using a set of labeled data, i.e. the training examples have an input (data) and a desired output (label or target value).
The aim of supervised learning is to learn a function that maps inputs to outputs based on training examples, so that the model is able to make accurate predictions on new, unseen data.
Some examples of supervised learning are Classification (predicting a class or category), Regression (predicting a numerical value), Anomaly Detection (identifying unusual instances), among others.
Unsupervised Learningo
In unsupervised learning, the algorithm is trained on a set of unlabeled data, i.e. there are no desired outputs provided. The main objective is to discover structures, patterns or groupings in the data.
The aim is to explore the hidden structure in the data and find insights, such as groups of similar items (clustering) or dimensionality reduction for data visualization or compression.
These include Clustering, Dimensionality Reduction (PCA, t-SNE), Rule Association (discovering relationships between items in a transactional dataset), among others.
Reinforcement Learning
Reinforcement learning is a paradigm in which an agent interacts with an environment and learns to make sequential decisions to maximize a cumulative reward over time.
The goal is to learn a policy (strategy) that allows the agent to take actions that optimize its reward over multiple decision stages.
For example, games (such as chess or Go), autonomous robotics, navigation of autonomous vehicles, control of dynamic systems, etc.
6 Benefits of Machine Learning for Business
The benefits of Machine Learning are numerous, especially in the business world. It can help companies predict trends, optimize processes, increase efficiency and, most importantly, improve the customer experience.
1. Trend forecasting
Machine Learning makes it possible to analyze large volumes of historical data to identify patterns and trends that may not be easily discernible to the naked eye.
This is especially useful for predicting future events, such as market demand, consumer behavior, price fluctuations or even weather events. These forecasts can help companies make informed and strategic decisions.
2. Valuable insights
Machine Learning can extract valuable insights from complex data. It is able to identify correlations, relationships and hidden information in data sets, allowing organizations to make more data-driven decisions.
These insights can help optimize operations, improve the effectiveness of products and services and even identify new business opportunities.
3. Efficiency
Automating routine tasks and complex processes is one of the main advantages of Machine Learning. It can automate data analysis, email sorting, chatbot customer service, among many other tasks.
This automation of tasks not only saves time, but also reduces human errors and operating costs.
4. Adaptability
Machine Learning models are able to adapt to changes in data and the environment. This means that they can learn from new data and adjust their predictions or decisions accordingly.
Adaptability is essential in dynamic scenarios such as finance, marketing and cyber security.
5. Algorithmic Company
Implementing Machine Learning in an organization can turn it into an "algorithmic company". This means that decision-making at all levels is driven by insights and predictions based on data, rather than intuition or fixed rules.
This can improve the organization's agility and its ability to innovate.
6. Better performances
Machine learning can improve performance in various tasks. For example, in computer vision, deep learning models have achieved human performance in image recognition tasks.
In medicine, machine learning systems can help identify diseases at an early stage. In finance, they can optimize investment portfolios. In short, Machine Learning can help achieve superior results in a wide range of domains.
Difference between Machine Learning and Big Data
Machine Learning and Big Data are two interrelated concepts that play crucial roles in the information age. However, they are distinct in terms of focus, objectives and application. Check out the main differences between them!
Machine Learning is a subfield of artificial intelligence that focuses on building algorithms and models that allow automated systems to learn and make decisions based on data.
The main focus of ML is the development of techniques that allow computers to improve their performance on specific tasks as they gain experience with data.
Big Data refers to the management and analysis of extremely large and complex data sets that exceed the processing capacity of traditional systems. The central focus of Big Data is dealing with the volume, variety, speed and veracity of data.
Its main objective is to extract meaningful information and insights from large volumes of data, often unstructured, for purposes such as strategic decision-making, identifying trends, personalizing products and services, detecting fraud, among others.
We can say that Machine Learning and Big Data often complement each other. The ability to process large volumes of data is essential for feeding Machine Learning models with enough information to learn and make accurate decisions.
Difference between Deep Learning and Machine Learning
The difference between Deep Learning and Machine Learning lies in the depth and complexity of the models and techniques involved in each approach.
Both are subfields of AI that focus on the ability to learn from data, but they differ in terms of architecture, application and depth of learning. See these differences in detail!
Machine Learning is a field of AI that focuses on developing algorithms and models that can learn and improve performance on specific tasks based on data. The focus is on building models that can make predictions or decisions without being explicitly programmed.
And Deep Learning is a subfield of Machine Learning that focuses on deep neural networks, which are models with multiple layers of processing. The main focus of Deep Learning is to learn hierarchical and complex representations of data, allowing models to automatically capture relevant features.
So we can say that Machine Learning is suitable for simpler, more straightforward tasks, where the relationship between inputs and outputs can be modeled relatively simply. Deep Learning shines in complex and abstract tasks, where understanding subtle characteristics is key.
Practical Examples of Machine Learning in Marketing
The application of Machine Learning in marketing has become increasingly common to optimize strategies, improve audience segmentation and increase ROI. Check out some practical examples!
Search Engine Optimization (SEO)
- Keyword analysis: Machine Learning can be used to identify trends and patterns in search queries. This helps in selecting the most relevant keywords and optimizing content to rank better in search engines.
- Content recommendations: ML algorithms can suggest topics and keywords based on user preferences, helping to create content that appeals to a wider audience.
- Detection of spam and low-quality content: ML can be used to automatically identify low-quality content or spam on websites, improving the overall quality of content and ranking in search results.
Content Marketing
- Content personalization: Machine Learning can analyze user behavior and interaction history to provide highly personalized content recommendations on websites, blogs and newsletters.
- Content generation: ML algorithms can be used to automatically create titles, descriptions and even entire articles based on topics and input data.
- Content performance analysis: Machine Learning can help identify which types of content and formats work best with different audience segments, allowing for more effective allocation of resources.
Paid Media
- Real-time bid optimization: ML algorithms can automatically adjust bids in ad campaigns, taking into account factors such as conversion history, location and the user's device.
- Advanced audience segmentation: Machine Learning can identify user profiles most likely to convert and target ads to these specific groups, saving resources and improving ROI.
- Fraud detection: ML algorithms can detect fraudulent activity in paid media campaigns, such as invalid clicks, helping to save money and improve the accuracy of metrics.
Social networks
- Sentiment analysis: Machine Learning can automatically analyze sentiment in social media posts, allowing companies to understand the public's perception of their brand and products.
- Audience segmentation: ML algorithms can segment social media followers based on interests, behaviors and demographics, allowing for more targeted campaigns.
- Trend and viral detection: Machine Learning can identify emerging trends on social networks, allowing companies to take advantage of engagement and marketing opportunities in real time.
Qualifying Leads
- Lead scoring: Machine Learning can assign scores to leads based on their profile and behavior, identifying qualified leads, those most likely to convert into customers.
- Automatic classification of leads: ML algorithms can automatically categorize leads based on predefined criteria, helping the sales team to prioritize their efforts.
- Automating follow-ups: Based on the behavior of leads, ML can automate personalized follow-up actions, such as sending emails or invitations to webinars.
Key Machine Learning Trends for the Future
Machine Learning is constantly evolving and promises to bring significant innovations in the future. Discover the main trends we can expect!
Increased Machine Learning (AutoML)
AutoML refers to the automation of previously challenging tasks in the Machine Learning lifecycle, such as data pre-processing, model selection and hyperparameter tuning. As organizations look to implement Machine Learning in a variety of contexts, the demand for AutoML will grow.
In addition, AutoML will allow domain professionals who are not Machine Learning experts to develop effective models more easily. AutoML platforms and tools will continue to evolve to make the model development process more accessible and efficient.
Expanding Federated Learning
Federated Learning is an approach that allows Machine Learning models to be trained on distributed data without this data needing to be shared centrally. This is crucial for data privacy and security, especially in highly regulated sectors such as health and finance.
In the future, we will see a significant expansion of federated learning, with more companies adopting this approach to train models on large volumes of sensitive data, without compromising privacy.
Growth of Machine Learning Explainable
As Machine Learning models become more complex, the need to understand why they make certain predictions or decisions becomes crucial, especially in critical applications such as healthcare and justice.
Explainable Machine Learning (Explainable AI) will focus on making models more transparent and able to provide clear justifications for their actions. This not only improves confidence in the model's decisions, but is also essential for complying with strict regulations.
Wider Use of Deep Neural Networks
Deep Neural Networks, such as convolutional and recurrent networks, have driven significant advances in computer vision, natural language processing and other domains.
It is expected that these architectures will continue to evolve and be applied in an ever wider range of applications. For example, in medicine, deep neural networks can be used for more accurate diagnoses, and in mobility, they can play a crucial role in autonomous vehicles.
Deeper Integration with the Internet of Things (IoT)
The combination of Machine Learning and the Internet of Things (IoT) is a growing trend. IoT devices collect data in real time from sensors and other devices, and Machine Learning can be used to analyze this data in real time, enabling automated and efficient decision-making.
This is especially relevant in applications such as smart cities, manufacturing, precision agriculture and healthcare, where IoT and Machine Learning can improve efficiency and people's quality of life.
Conclusion
As you can see, Machine Learning has become an indispensable tool for companies, not only because it allows them to understand their data, but also to use it to predict trends, optimize processes and improve the customer experience.
In the world of marketing, Machine Learning has become increasingly relevant, optimizing strategies and ensuring that professionals make increasingly accurate decisions - based on data.
In an increasingly competitive business environment, incorporating Machine Learning into marketing strategy is no longer an option, but a necessity. It is therefore essential that companies not only adopt Machine Learning, but also continue to explore and experiment with its possibilities.
To find out more about topics related to Machine Learning and Artificial Intelligence, we invite you to read our article on Artificial Intelligence Robots, in which you will understand how an AI bot works and its contribution to business.