Trends in data and AI are set to significantly transform businesses. PwC predicts that AI will contribute more than $15 trillion to the global economy by 2030.
In addition, Grand View Research projects annual growth of 37% in the global artificial intelligence market between 2023 and 2030, indicating an ongoing technological revolution.
Data analysis, driven by AI, is becoming crucial to business success. The integration of these technologies is changing the way organizations extract and use insights from data.
Companies that adapt quickly to these changes will be better prepared to seize new opportunities and face the challenges of the digital age. In this article, we will explore the sectors most impacted, the expected benefits and how to incorporate these technologies to remain competitive.
Difference between Traditional AI and Generative AI on Data
Traditional AI and Generative AI represent different approaches to artificial intelligence. Traditional AI focuses on specific tasks such as classification and prediction, dealing with structured data and producing predictable results. Generative AI creates new and original content from learned patterns, working with unstructured data and generating varied and creative results.
A practical example of this difference is the analysis of behavioral data. Suppose ChatGPT-4, a Generative AI, analyzes a report with 1000 participants.
Rather than just providing statistical projections, it offers a more human perspective, highlighting trends such as the transformation of solitary activities into social experiences, the impact of the pandemic on personal habits, the preference for hybrid working models and the increase in mental health awareness.
This analysis illustrates how Generative AI can go beyond numbers, offering contextualized insights and a narrative that captures social and emotional nuances, something that Traditional AI could hardly achieve.
1. Advances in Natural Language Processing (NLP)
Natural Language Processing (NLP) is experiencing significant advances in 2024, revolutionizing the way machines understand and interact with human language. According to Statista, it is estimated that the NLP market will be worth more than US$40 billion by 2025, reflecting its growing importance and applicability.
Recent advances:
Large Scale Language Models (LLM): evolutions of models such as ChatGPT-4 are providing unprecedented capabilities in text comprehension and generation;
Enhanced Contextual Understanding: mindfulness techniques and transformers enable a deeper understanding of the context and nuances of language;
Multilingualism and Real-Time Translation: improvements in machine translation and the processing of multiple languages simultaneously;
More accurate Sentiment Analysis: ability to detect emotional subtleties and sarcasm in texts, improving feedback analysis and customer interactions;
Natural Text Generation: advances in the creation of textual content that is practically indistinguishable from that written by humans;
More natural conversational interfaces: chatbots and virtual assistants with more fluid and contextual conversational skills.
2. Automated Machine Learning (AutoML)
Automated Machine Learning (AutoML) is emerging as a transformative technology in 2024, democratizing access to data analysis with AI. Here's why.
- Data preparation: AutoML automates data cleaning, transformation and pre-processing, reducing errors and preparation time for analysis;
- Feature Selection: automatically identifies the most relevant features in the data for the analytical problem in question;
- Algorithm selection: tests various machine learning algorithms to find the most suitable one for analyzing the specific data set;
- Hyperparameter Optimization: automatically adjusts the model's parameters to maximize its performance in data analysis;
- Model Evaluation and Comparison: compares different models generated, selecting the most effective for the desired analysis;
- Implementation and Monitoring: facilitates the implementation of the chosen data analysis model and monitors its performance on an ongoing basis.
3. Data as a Service (DaaS)
DaaS allows companies to monetize and provide access to internal data insights as a service. It is an information supply and distribution model in which data is made available to customers via the internet. Check out its benefits:
- Access to High Quality Data: companies can access comprehensive, enriched and up-to-date data sets from third parties, without the need to collect and maintain them internally;
- Greater Variety of Data: DaaS expands the types of data available for analysis, including structured, unstructured, third-party, public and private data;
- Valuable Insights: in addition to raw data, DaaS also offers access to sophisticated analysis and AI-based insights already processed by experts;
- Cost reduction: eliminates the need for significant investments in data infrastructure and specialized in-house teams for data management and analysis;
- Scalability: allows companies to scale their analytical capabilities more quickly, accessing more data as needed, without hardware or software limitations;
- Real-time access: many DaaS providers provide access to continuously updated data and insights, enabling real-time analysis.
4. Preparing your Company for the Future with a Focus on Data and AI
As data analysis and AI continue to evolve rapidly, it is crucial that companies prepare themselves adequately to remain competitive and take advantage of emerging opportunities. That's why we've put together some implementation strategies. Check them out!
Assessment and Planning: Start with a comprehensive assessment of your company's current data and AI capabilities. Identify gaps and areas of opportunity. Develop a strategic roadmap aligned with business objectives;
Data Infrastructure: invest in a robust and scalable data infrastructure. Prioritize data quality, security, accessibility and integration of the world's largest AIs in one place. Get to know Tess AI!
Strategic Partnerships: consider partnerships with technology providers, such as innovative startups. These collaborations can accelerate the adoption of AI and bring in external expertise;
Talent development: invest in the qualification and retraining of your workforce. Create internal training programs and encourage continuous learning in data science and AI.
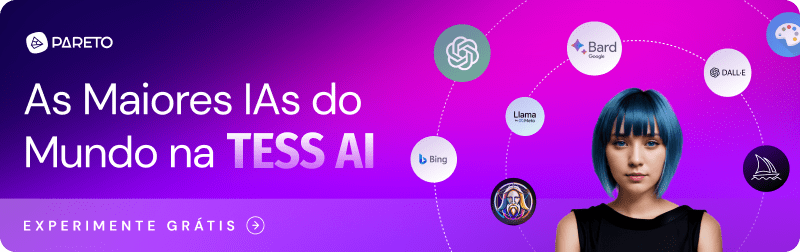
5. AI Tools for Data Analysis
With the growing adoption of AI for data analysis, various platforms and tools are emerging to allow companies to exploit the full potential of this technology. Here are some of the main options:
Luzmo: this embedded analytics platform makes it easy to add analysis with minimal effort. Its main innovation is to allow end users to generate interactive analytical dashboards through natural language prompts, using generative AI.
Tableau: Tableau's business intelligence suite is incorporating more and more AI features. It makes it possible to carry out forecasts, "what if" scenario simulations, statistical modeling and other data science techniques directly on the platform.
Microsoft Power BI: Microsoft BI has enriched its capabilities with AI. It can apply natural language processing to data, performing sentiment analysis, key phrase extraction, language detection and more.
KNIME: this open source tool offers a visual and intuitive interface for data science. It allows you to design workflows, train and apply machine learning models for data analysis in a simplified way.
6. New demands for data and AI skills
The rapid advance of data analysis and AI is drastically transforming the job market, creating an urgent demand for specialized skills and challenging companies to develop and attract suitable talent. Discover the most sought-after skills:
- Proficiency in Natural Language Processing;
- Machine Learning;
- Data Science;
- Proficiency in AI Ethics;
- Cloud Computing;
- Programming in Python/R;
- AutoML;
- Ability to translate technical knowledge into business value.
7. Automation and Job Creation in Data and AI
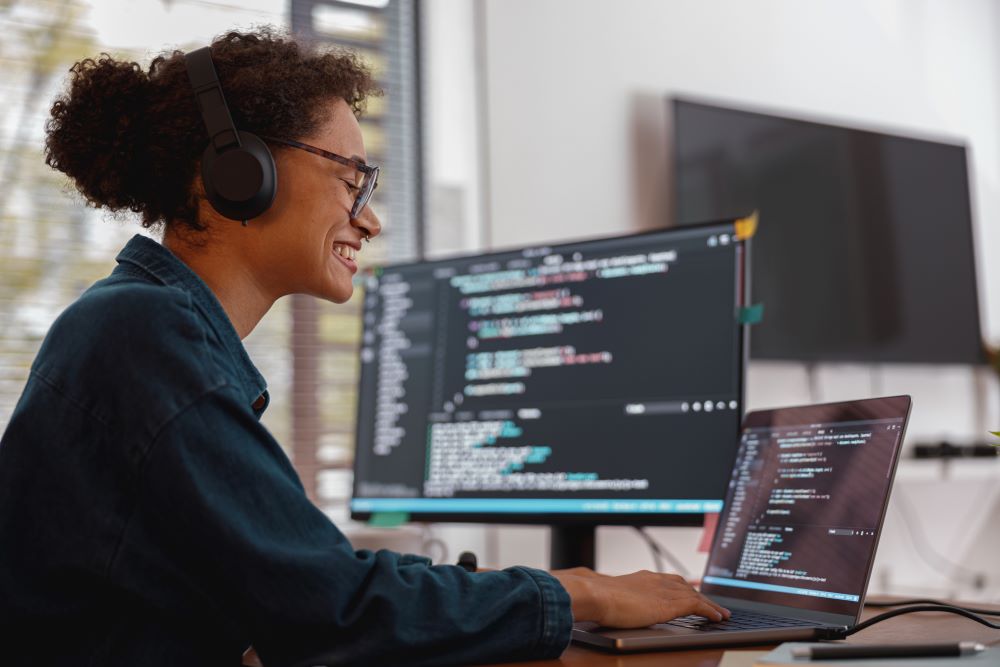
While automation may replace some traditional jobs, it is also generating a vibrant ecosystem of new opportunities, driven by data analysis with AI.
These new roles require a combination of technical, creative and interpersonal skills. According to the World Economic Forum, by 2027, approximately 23% of jobs will be transformed, with automation with AI creating around 69 million new jobs, while 83 million jobs will be eliminated.
New Emerging Functions:
- Machine Learning Operations (MLOps) engineers, as predicted by the Data Hackers Podcast;
- AI ethics experts;
- Prompts Engineers;
- Data Scientists;
- Experts in Generative AI;
- "AI translators" - professionals who connect technical experts in AI with other areas of the company.
8. Ethics and Regulation in AI for Data Analysis
As AI becomes more prevalent in data analysis, ethical and regulatory issues gain increasing prominence. Check out the evolution of regulations below:
- European Union: leads the way with the AI Act, setting strict standards for high-risk AI systems.
- United States: adopts a sectoral approach, focusing on data privacy and non-discrimination.
- China: implements regulations for AI algorithms, emphasizing national security and content control.
Best Practices for Companies:
- Establish AI ethics committees and implement trustworthy AI frameworks;
- Invest in continuous training on AI ethics for data and AI teams;
- Auditing biases and impacts of data analysis algorithms;
- Maintaining compliance with constantly evolving regulations.
Adopting ethical and responsible governance practices in AI for data analysis is a strategic imperative. Reliable and transparent analytical systems increase the trust of customers, employees and other stakeholders in the long term.
Conclusion
With innovative technologies such as natural language processing and automated machine learning, you will have the opportunity to gain competitive advantage through deep insights extracted from data.
However, in order to take full advantage of the potential of data analysis with AI, companies need to be prepared by evaluating their capabilities, infrastructure, talents and ethical practices.
To accelerate your adoption of data analysis with AI and access external expertise, explore Tess AI, Pareto's Generative AI platform.
With the best technologies on the market integrated, this collaboration can boost your analytical transformation with cutting-edge solutions. Try Tess AI for 7 days with a satisfaction guarantee!
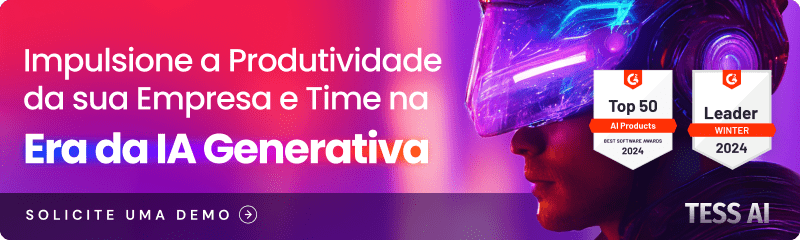