After creating landing pages, choosing creatives, writing emails, determining button text and CTAs, how do you predict people's interactions and the outcome of your campaign? Do you just rely on your intuition?
This is when an A/B test is essential! It reveals which version of your campaign has the best performance and, consequently, the highest conversion rate.
Read on to find out how to plan the entire A/B testing process. At the end, we'll show you how simple it is to run an experiment with Pareto Ads!
What is A/B Testing?
AB Testing is a methodology for comparing two versions of a webpage, app, audience or advertisement to determine, based on a comparison variable, which one gives the best result.
It's also known as split testing, where you conduct a marketing experiment in which you divide your audience to test various variations of a campaign and determine which performs best.
Every A/B test should be based on hypotheses formulated on the basis of your brand's knowledge and differentials. Ideally, you should establish a schedule of tests you want to carry out. This way, the information is tested in parts.
Why are A/B tests effective?
The AB Test's main objective is to generate an increase in performance, such as:
- Increase the Conversion Rate;
- Reduce the site's Bounce Rate;
- Increase the expectation of Sales/Leads among Audiences.
You can separate the tests by marketing funnelreaching audiences at different points in their buying journey. See in the image below that we can start by testing ads, then Landing Pages and finally welcome emails:
What to Test?
Check out the A/B testing possibilities separated according to the stages of the marketing funnel:
Announcements
You can test ad variations, such as changing the copywright, making changes to the creative (in the case of an image), replacing the color of a CTA or even the button phrase.
- Image;
- Text;
- CTA buttons;
- Public;
- Format.
Landing Page
The universe of LP's is even wider. You can change the main banner, increase the number of CTA's on the page, change the color, replace fields in a form, show/hide the price of a product service.
- Titles;
- Intertitles;
- CTAs;
- Images;
- URL;
- Form fields;
- Product description;
- Visual elements .
It has similar aspects to the ad, but in this case we already have the customer's e-mail address. In this way, it is assumed that there has been a first contact with your company (which is why we are at the bottom of the funnel stage).
You can then change the communication of the email, test the use of category or product images, CTA's, redirect links and more variations.
- Titles;
- Intertitles;
- CTAs;
- Images;
- Elements;
- Date and time of dispatch.
How Does Testing Impact Performance?
For a B2B company, the main focus is to increase the volume of qualified leads. For an e-commerce business, the ultimate goal is to increase the volume of sales and generate more revenue.
However, in both cases, A/B testing can resolve the following points:
a. Improve the user experience;
b. Improve traffic qualification;
c. Reduce the bounce rate;
d. Make controlled changes to the site with low risk;
e. To have statistically reliable results.
A/B Test Example
A statistical test should have a rational meaning, and not be a random attempt. In this case, we define a hypothesis based on our knowledge of the business. The ideal is to find something that differentiates you from your competitors, so that this allows you to improve the metric you have chosen as your objective.
Here at Pareto, we define the best metrics to focus on, depending on the stage of your campaign funnel and the volume of data in your account. If your company has already passed the learning stage, it will have accumulated a lot of information, so we can carry out a more advanced test.
We have developed a standard template to make it easier to create a hypothesis, just fill in the gaps:
IF my ad varies .................................... SOON I hope to optimize my results WHY .....................................
Here's an example of an ad variation:
When the ads are published, there is a 50% impression frequency for each of the versions: version A and version B of the ad and the results will be monitored until the end of the test period.
The more data collected, the more accurate the decisions. Once enough data has been accumulated from the original ad and the variation, a comparison is made and then we determine which one performed better.
The A/B Test can be created with the help of the Google Ads tool, which allows you to experiment with ads. At the end of the test, we'll have 95% confidence that the ad was the winner. Then, a suggestion is made to pause the "losing" ad in order to keep only the "winning" one.
Here's an example of an A/B test result in a campaign. We can see a comparison of clicks, CTR, Cost, Conversions and various other metrics.
This will save money, as a more efficient ad will generate more qualified clicks, leading to a higher conversion rate. In addition, the quality index will be better, allowing keyword bids to be reduced without jeopardizing the position ranking.
How to do an A/B test?
AB testing can be separated into five stages: Research, Observation and Hypothesis Formulation, Variation Creation, Execution and Result Analysis.
1. Research
The research consists of analyzing current data. It seeks to answer the main question: "How am I today?".
It may seem simple, but for each test, we have a data platform with different metrics to analyze. Creating an A/B test without proper research could cause minimal performance impacts.
Therefore, applying the Pareto principle (80/20) is important to align the test strategy with the objective you want to achieve.
For each test we have a specific targeting platform:
- Google Analytics - Test on the site as a whole (e.g. an LP);
- Facebook Ads - Audience, Ads, Positioning Test;
- Google Ads - Audience and Ads Test;
- Firebase - Application Test
2. Observation and Hypothesis Formulation
This is the most critical stage! Creating a test without a hypothesis is like going on a sea voyage without a compass. In other words, you're traveling aimlessly, with the uncertainty of getting anywhere.
Before we continue, let's take a closer look at the concept of hypothesis. A hypothesis is a statement created in simple language that must be challenged.
Let's look at an example of Hypothesis applied to a Pareto advertisement:
Hypothesis: Ads from Pareto's Brand campaign get more clicks when the term Free Trial is used in Headline 2.
3. Creating the Variation
Based on the hypothesis, we will put the statement to the test by creating a variation group and a control group (the original).
It is possible to create a test with multiple variables, but the more simplified the test, the shorter its duration. And the more effective it will be in determining the real reason for the performance improvement! See this other article for an explanation of Statistical Ad Experiments.
Continuing with the Pareto example:
Control Group:
Experiment Group:
4. Testing
Once the variation has been created, we have to put the test into practice!
It is important to remove any biased variables applied during the test. For example, when testing the ad above, ideally ensure that the number of impressions or the investment for each variation remains as close as possible.
5. Analysis of results
Once the AB Test is complete, it's time to analyze the main results generated. Important performance metrics should be evaluated, such as: cost, clicks, CTR, conversions, CPA or ROAS, among others.
Let's take an example:
Control Group
Cost: R$4,000
Clicks: 3,785
CTR: 4.25%
Conversions: 112
CPA: R$35.12
Group Variation
Cost: R$4,050
Click: 4,003
CTR: 5.12%
Conversions: 96
CPA: R$42.18
What's next? What's the best ad?
Following the hypothesis tested above, that the term "Free Trial" would increase the number of clicks, the winning ad is Variation. Because both the number of clicks (+5.7%) and the CTR (+20.5%) are higher when we have "Free Trial" in Headline 2 of the ad.
However, analyzing in terms of conversion, it is possible to see that the number of conversions fell from one variation to the next, with a 34.6% increase in CPA.
So, in terms of clicks, the example does indeed perform better. In terms of conversions, however, the answer is completely the opposite. Now just apply the test to the ads in your account!
How do you measure your results with Pareto Ads?
At Pareto Ads, we have a One Click optimization card specifically for A/B testing ads!
So whenever two ads are running to the same audience, our algorithm automatically runs a test between them and is able to report the results when it finds a statistically significant result.
To do this, you need to have a sufficient volume of data for the metrics to be relevant to this conclusion. We recommend applying experiments with over 80% statistical confidence for the best results.
See the example below of how the Ad Experiment Card will appear for both Google and Facebook:
The algorithm identifies the test being applied to your Google Ads account, evaluating the account's ads in that specific campaign and ad group.
In the same One Click, you can see which test was carried out and the results! In this case, the main variation is the Headline 2 change.
The test metrics are shown, with the statistical result and the winning and losing ad. In this case, the evaluation metric is CPA (Cost per Acquisition).
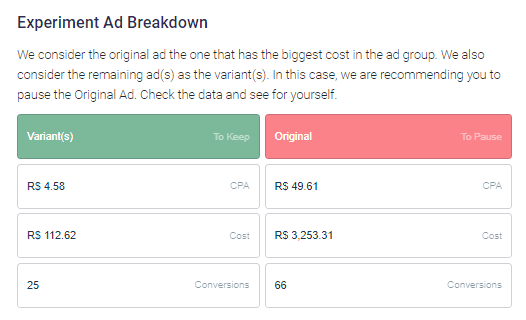
And something that makes it incredibly easy to manage: Pareto Ads runs the test with just one click on the "Execute" button. This way, the losing ad is automatically paused in your account!
Now, let's look at an example of One Click Ad Experimentation on Facebook Ads. In this case, the algorithm is evaluating the creatives that are running for the same audience. We also have all the relevant information, as well as the statistical significance and previous creatives that were running for decision making.
In this article, you learned more about the important steps in setting up an A/B Test and how Pareto helps you apply it to your Google Ads and Facebook Ads.
Once an A/B test has been completed, you can continue with the testing schedule, always with the aim of further improving the quality of the ad (or the variable that was tested). New information can be tested successively. This virtuous cycle is essential for optimizing campaigns.
A/B testing is an excellent opportunity to save money and make your campaigns even more effective. But remember that if it's not set up properly, instead of optimizing and saving your campaigns, it can cause losses by allocating money to non-productive tests.
Also, pay attention to the statistical reliability of your A/B test results, set the deadlines appropriately so that the data collected is sufficient. That way, the best and most reliable decisions can be made for your campaigns.